Abstract
Cortical development is a highly regulated process that relies on the precise control of the cell cycle to generate the appropriate number and types of neurons. Genetic or environmental perturbations in the cell cycle of neural progenitor cells can influence their proliferation and fate specification with such dysregulation postulated as the primary cause of cortical malformations, such as prenatal microcephaly. To gain a deeper understanding of the molecular mechanisms underlying the relationship between the cell cycle and differentiation, I intend to combine single-cell transcriptomics (scRNA-seq) methods with well-validated cell tracing techniques to comprehensively characterize cellular states across various developmental time periods. More specifically, our aims are as follows:
(Aim 1): Develop feature barcoding methods to integrate readouts from complementary cell tracing techniques into scRNA-seq assays. Unlike existing methods that artificially separate cell populations into bins, our approach will directly quantify the amount of tracer in each cell, significantly increasing resolution and enabling the use of more refined statistical models.
(Aim 2a): Characterize the transcriptional states resulting in specification of neuronal subtypes as a function of the number and rate of neural progenitor divisions.
(Aim 2b): Investigate the effect of alterations in mitotic length on changes in differentiation using heterochronic mouse models. These combined approaches will enhance our understanding on the spatiotemporal heterogeneity of neural progenitor fate determination.
Specific Aims (1 page)
Neurogenesis requires the precise orchestration of proliferation and differentiation, both of which are intimately related to the cell cycle. This relationship is evident in the brain, where the cell cycle tends to lengthen on average as brain development progresses (Ponti et al., 2013). Moreover, acute lengthening of the cell cycle has been shown to disrupt neurogenesis, resulting in a reduced number of progenitor cells and altered cell fate specification, which are associated with neurodevelopmental and psychiatric disorders (Mitchell-Dick et al., 2020). Although the single-cell diversity of the developing cortex is becoming increasingly well characterized (Johnson et al., 2015), our understanding of how temporally progressing transcriptional states, particularly the cell cycle, generate the neuronal diversity remains limited. A comprehensive understanding of transcriptional signatures that control the proliferation and differentiation of neural progenitor cells can pave the way for valuable insights into cortical development and its associated disorders.
Cell tracking and labeling assays are based on the timed introduction of tracers, such as nucleoside analogs incorporation and fluorescent dye dilution. To integrate this information with the cell’s transcriptiomic state, a common approach involves sorting cell populations based on fluorescence intensity of tracers prior to single-cell sequencing (Lu et al., 2022). However, this method of artificially categorizing continuous cell populations into 4-6 bins inevitably leads to information loss and introduces bias (Trippe et al., 2022).
Instead, I will leverage feature barcoding techniques to simultaneously measure the quantity of tracers and the cell’s transcriptional state during single-cell sequencing. This approach eliminates tracer quantization and significantly improves the resolution of our analysis, which is essential for accurate statistical model fitting and data interpretation (Hyrien & Zand, 2008).
Aim 1: Develop a set of molecular tools to explore the mechanism of neural progenitor fate specification as a function of the cell cycle. This includes the integration of existing tracing tools, such as EdU pulse labeling, with scRNA-Seq through feature barcoding.
Aim 2a: Investigate how neuronal subtype specification is affected by key parameters of the cell cycle, particularly the number and rate of neural progenitor divisions. I will use the developed tools to assess the distribution of cell cycle rates during fate specification and how it relates to the cell’s transcriptional signature over multiple time periods.
Aim 2b: Demonstrate the effect of cell cycle perturbation on fate specification using genetic and pharmacological approaches. I will assess changes in the expression of fate-determining transcription factors and evaluate the transcriptional signature of altered fate decisions. From this, I will identify downstream effector genes of cell fate specification that are dependent on proper cell cycle timing.
Research Strategy (6 pages, including figures and tables)
Background and Significance (~1.5 pages)
Neurogenesis depends on the precise regulation of neural progenitor cell proliferation, cell cycle exit, and differentiation to generate a diverse array of neurons and glial cells in the correct time and location. Progenitor cell populations transition through various modes of division over developmental time periods. Initially, the progenitor pool expands through symmetric divisions, followed by patterning via differential growth rate during asymmetric and symmetric neurogenic divisions.
Furthermore, cell fate specification is known to be inextricably linked to the cell cycle in stem cells (Lange & Calegari, 2010; Pauklin & Vallier, 2013). In the brain, the G1 phase of neural progenitor cells is known to lengthen over the course of cerebral cortex development (Calegari et al., 2005). This happens as progenitor cells alter their competence to generate different cell types over time (Kohwi & Doe, 2013). The mammalian cortex’s signature six-layered structure is based on this process as it is formed by consecutive waves of neurogenesis with laminar fate of each neuron determined during the final S or G2 phase of the progenitors (Frantz & McConnell, 1996).
Consequently, it is unsurprising that disruptions to the cell cycle can have detrimental effects on neurogenesis. In humans, heterochronic mutations are associated with microcephaly (Doobin et al., 2016). Moreover, microcephaly secondary to Zika virus infection has been linked to mitotic catastrophe in neural progenitor cells (Rychlowska et al., 2022).
In addition to global alterations in cell cycle kinetics, local differences in cell cycle kinetics across neural progenitor populations are known to influence fate specification (Fabra-Beser et al., 2021). Single-cell analyses of neocortical progenitors have revealed molecular and cellular heterogeneity (Johnson et al., 2015; Yuzwa et al., 2017). While progenitors are known to produce heterogeneous neuronal populations, the mechanisms and timing of fate decisions remain to be elucidated (Llorca et al., 2019). A transcriptomic exploration of these rare cells during neural development can offer novel insights into the mechanisms governing progenitor fate specification and may reveal previously undiscovered therapeutic targets. Furthermore, by examining progenitor cells at the single-cell level, I can measure and analyze cell-to-cell variability, which can help uncover the sources of this variability and reveal its functional consequences during neural development.
This study will serve as both an experimental and computational training experience for me. I need to acquire a thorough understanding of molecular biology fundamentals in order to develop these assays. Additionally, analyzing single-cell transcriptomics data requires advanced computational and statistical methods. By performing both of these tasks, I can possess a comprehensive understanding of the project’s objectives and the underlying biological principles. This holistic approach enables me to identify potential optimizations and pitfalls during assay design and analysis, and helps to minimize any misinterpretation of results and accelerates the troubleshooting process when challenges arise.
Research Design and Methods (~4.5 pages)
Aim 1: Development of Cell Cycle Profiling Tools
To answer the questions described in the specific aims, we require a set of molecular tools that can provide temporal and spatial context to single-cell transcriptomes. I plan to develop a set of cell tracing tools that can be precisely quantified alongside the cell’s transcriptome via scRNA-seq without cell sorting. This approach also avoids quantizing the data, which is crucial for statistical model fitting (Hyrien & Zand, 2008). I have selected several labeling reagents based on their complementary capabilities.
CFSE
During development, neuroepithelial cells proliferate in the ventricular zone (VZ) that are adjacent to the ventricular lumen. A hallmark feature during early development is interkinetic nuclear migration, in which the nuclei are translocated according to the cell cycle phase, with the M phase occurring in the apical (luminal) zone while S-phase occurs in the basal (pial) zone (Kosodo et al., 2011). This translocation of cell mass allows us to directly and specifically label M-phase cells through an intraventricular injection of carboxyfluorescein succinimidyl ester (CFSE), a cell-permeable, amine-reactive variant of fluorescein. The dye’s short half-life and reactivity functionally restrict pulse-labeling to juxtaventricular cells (Telley et al., 2016). After an initial wash phase, the fluorescence signal intensity is diluted twofold after each cell division.
The dye’s short half-life and reactivity functionally restrict pulse-labeling to juxtaventricular cells (Telley et al., 2016). After an initial wash phase, the fluorescence signal intensity is diluted twofold after each cell division. Using this strategy, isochronic cohorts of VZ neural progenitor cells can be identified and tracked through their proliferation and differentiation. However, this fast kinetics also restricts labeling only juxtaventricular cells. Hence, this approach is the most useful for visualizing the migration profile of different progenitor cohorts.
EdU
Ethynyl uridine (EdU) is a nucleoside analog that is incorporated into the cell’s DNA during the S phase and is widely used for proliferation tracking. EdU is persistent through the cell’s life and gets diluted into its progeny, enabling us to track cells over long periods of time. The main advantage of EdU compared to CFSE labeling is that EdU can be introduced system-wide through an intraperitoneal injection, albeit at the cost of lower time resolution. To detect EdU, the ethynyl group can be reacted with an azide group in a simple CuAAC bioorthogonal reaction. Unlike earlier nucleoside analogs, such as BrdU, this requires no heat treatment for epitope unmasking. EdU’s downside includes its toxicity, as it can trigger mitotic arrest through the activation of DNA repair pathways (Ligasová et al., 2015). Thus, I plan to use f-ara-EdU, a significantly less toxic EdU variant, for our studies (Neef & Luedtke, 2011).
iCOUNT
The inducible cell division counter (iCOUNT) method relies on recombination-induced tag exchange (RITE) of endogenously tagged long-lived proteins to fluorescent proteins to track the number of cell divisions (Denoth-Lippuner et al., 2021). In this case, histone variant H3.1 is tagged with mCherry and switches to EGFP upon Cre activation. Then, every subsequent cell division reduces the amount of H3.1-mCherry by half, as new H3.1-EGFP is produced to refill the pool of histones. By comparing the ratio of intensity between mCherry and EGFP, I can infer the number of cell cycles since Cre activation. This approach allows us to accurately count the number of cell divisions through integrated signal normalization. As this is a Cre-based system, genetic-based labeling is also possible. The authors claim that this method can count up to 3-4 cell divisions in vivo. iCOUNT mice are now commercially available, and our collaborator, Dr. Dwight Bergles, already has them expanding in his colonies.
The inducible cell division counter (iCOUNT) method relies on recombination-induced tag exchange (RITE) of endogenously tagged long-lived proteins to fluorescent proteins to track the number of cell divisions (Denoth-Lippuner et al., 2021). In this case, histone variant H3.1 is tagged with mCherry and would switch to EGFP upon Cre activation. Then, every subsequent cell division would reduce the amount H3.1-mCherry by half as new H3.1-EGFP is produced to refill the pool of histones. By comparing the ratio of intensity between mCherry and EGFP, I can infer the number of cell cycles since Cre activation. This approach provides several benefits compared to previous methods, including integrated signal normalization and system-wide labeling. The authors claimed that this method can count up to 3-4 cell divisions in vivo. iCOUNT mice are now commercially available and our collaborator, Dr. Dwight Bergles, already has them expanding in his colonies.
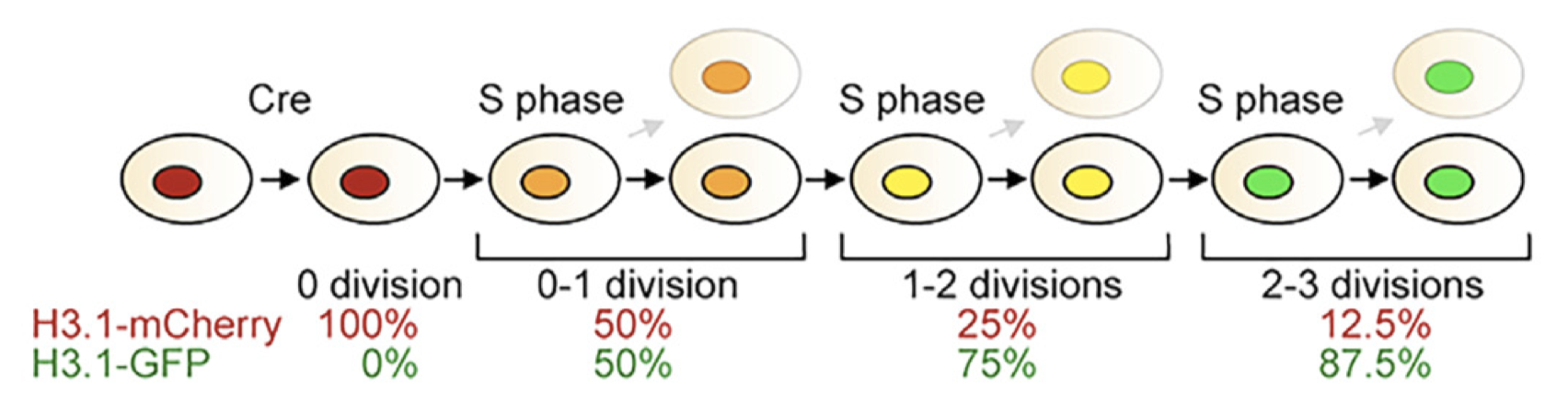
Figure 1b from (Denoth-Lippuner et al., 2021)
Combining Labeling with Transcriptomics
The study of neural progenitors has relied heavily on these labeling strategies. However, their utility could be significantly enhanced if we could precisely combine the labeling readouts with transcriptome-wide measurements. Current studies utilizing these labeling strategies are generally limited to imaging in conjunction with immunofluorescence labeling (Telley et al., 2016), cell sorting (Lu et al., 2022), and a limited set of genes using FISH (West et al., 2022). In order to combine these labeling strategies with scRNA-Seq methods, we need to label these tags with oligonucleotides (Black et al., 2021; Stoeckius et al., 2017). For CFSE and iCOUNT, I will use oligonucleotide-conjugated antibodies to FITC (Good et al., 2019), EGFP, and mCherry, respectively. Linking EdU to an oligonucleotide has proven more technically challenging, as we do not want to use antibodies, as these necessitate harsh epitope unmasking. Instead, I designed an assay in which biotin azide is reacted with EdU and subsequently detected with oligonucleotide-conjugated streptavidin. CFSE can be labeled with anti-FITC antibodies with good efficacy.
I plan to use sci-RNA-seq3 (Martin et al., 2022), a cost-efficient method that would allow us to scale our analysis to larger cell numbers and conditions. Afterwards, I will use the software I wrote along with published software tools, such as kallisto, to run the processing pipeline. Differential gene expression analysis can be done using the likelihood ratio test comparing the expression value between cells in different conditions. Heterogeneity can be assessed through gene set enrichment analysis techniques, particularly linear additive models.
Validation
I will perform validation of these labeling techniques using in vitro cell culture experiments with the HEK293T and Jurkat cell lines. To establish the ground truth of cell cycle parameters, I will employ the Fluorescent Ubiquitination-based Cell Cycle Indicator (FUCCI) (Bajar et al., 2016) to track the cell cycle in a live cell imaging experiment. This FUCCI system has been established in the lab, and clonal populations of cell lines have been generated. To determine the sensitivity of the detection of the change in rate, I will pulse cells with EdU/CFSE alongside pharmacological agents that perturb the cell cycle, such as hydroxyurea and nocodazole. I will collect the cells for sequencing after several chase intervals. This will be done in concordance with the tricycle algorithm, which phases the position a cell is in its cell cycle from its transcriptional state (Zheng et al., 2022). Tricycle provides substantially higher resolution compared to the standard method of artificially binning the cell cycle into four phases and analyzing them separately.
Aim 2a: Investigate how specification of neuronal subtypes is affected by key parameters of the cell cycle
Previous research has identified Sox9 as a mediator of cell cycle length in a subset of radial progenitor cells exhibiting reduced neurogenic behavior (Fabra-Beser et al., 2021). These findings align with other studies employing pharmacological approaches to slow the cell cycle (Mitchell-Dick et al., 2020). We aim to build upon these results by conducting a transcriptome-wide analysis of radial progenitor cell populations and their temporal behaviors using the methods outlined in Aim 1. By examining mice from various developmental timepoints between E10.5 and E15.5, I will identify differentially expressed genes correlating with cell cycle position and other cell cycle parameters. Additionally, our tools’ high-resolution tracing capabilities will enable parallel examination of heterogeneous populations, allowing for the identification of key differences in their transcriptomic signatures. As a validation step, I plan to verify whether Sox9 is differentially expressed across apical progenitors as a function of cell cycle rate.
Aim 2b: Demonstrate the effect of cell cycle perturbation on fate specification using genetic and pharmacological approaches
Cell cycle dynamics’ role in neurogenesis becomes evident through genetic or pharmacological perturbation. In humans, heterochronic mutations are associated with microcephaly (Doobin et al., 2016), while Zika virus-induced microcephaly is linked to mitotic catastrophe in neural progenitor cells (Rychlowska et al., 2022). Experimental settings have demonstrated mitotic defects resulting from gene mutations, such as Magoh (Sheehan et al., 2020). I plan to utilize a mouse line familiar to our lab, the Pantr2 KO mouse (Augustin et al., 2022). Knocking out Pantr2, a long non-coding RNA, alters cell cycle dynamics along with fate specification. Using tools developed in Aim 1, I intend to investigate the affected regulatory networks that lead to these alterations in detail.
Data analysis
I will reconstruct cellular histories by integrating transcriptomic data with tracer data. Cell cycle kinetics can be inferred using the CycleFlow algorithm (Jolly et al., 2022). By employing the tracer as a covariate, I will initially perform regression analysis to identify differentially regulated genes in cells exhibiting varying cell cycle kinetics. This approach will enable us to implicate known pathways in specific cellular contexts and uncover new functional gene groupings that affect distinct neuronal subtype fate specification in relation to prolonged mitosis’s impact on microcephaly and related developmental disorders.
Moreover, I will leverage my lab’s expertise with latent space representations to develop analytic approaches that better represent complex biology compared to marker gene-based techniques. These analyses will facilitate the discovery of new functional gene groupings influencing the fate specification of distinct neuronal subtypes concerning the effects of extended mitosis on microcephaly and associated developmental disorders.
Anticipated problems that might arise and alternative plans to accomplish the specific aims if these problems arise
I have obtained preliminary data demonstrating the in vitro success of these methods through oligonucleotide-tagged EdU detection using sci-RNA-Seq3 and flow cytometric detection of oligonucleotide-conjugated antibodies to CFSE. By having multiple tracers, we lower the risk of all assays failing. The remaining challenge is adapting the method to in vivo conditions. However, as both EdU and CFSE are widely used, I do not expect significant difficulties. If problems do arise, I can connect direct fluorescent intensity to the transcriptome using imaging-based multiplexed FISH techniques.
Tentative sequence for the investigation
Year 1 (Present - December 2023)
I plan to complete the primary development and in vitro characterization of assays described under Aim 1.
Year 2 (January - December 2024)
After gaining broad understanding of the assays and their properties, I will begin Aim 2A and 2B of my research plan. I will study and infer cell cycle parameters in the embryonic mouse brain as described in Aim 2A. Afterwards, I will then move to Aim 2B and extend my study to Pantr2 mice, a heterochronic mouse line, to investigate the mechanisms in which the cell cycle is altered.
Year 3 (January 2025 - ~June 2025) Anticipated year of graduation
I will rigorously analyze the data collected from all of my aims to begin to draw conclusions and develop a predictive mathematical model as a function of the cell cycle. I believe I will discover differentially regulated genes in different progenitor population as a function of their cell cycle kinetics.